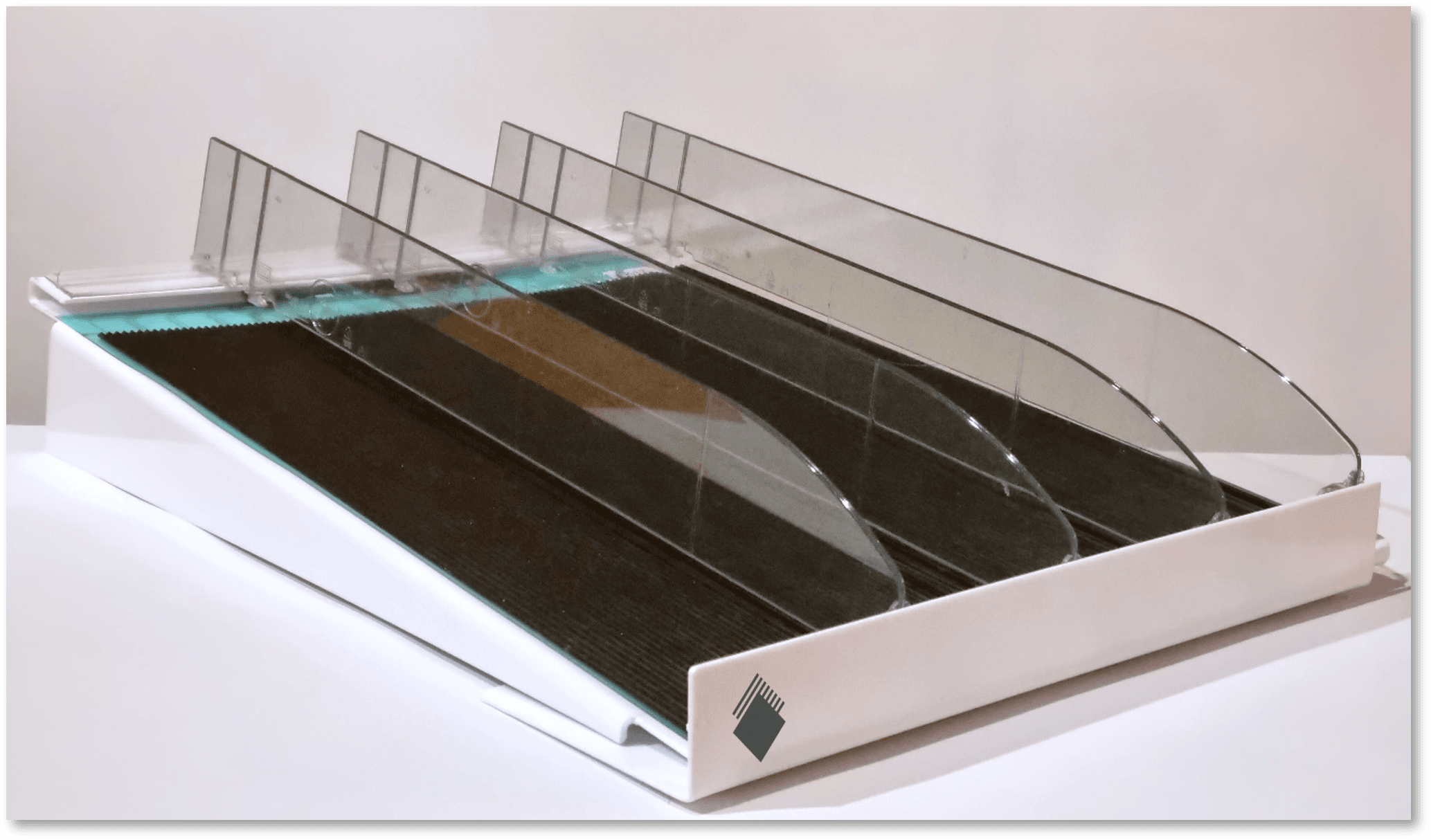
Making Sense of Sensors
MOOS follows an unconventional path towards 10-100x better sensor TCO
Rethinking Sensor Technology: Harnessing the Power of AI
Recent breakthroughs in AI have created huge disruptive potential for rethinking the development and deployment of sensors. Traditionally, sensor manufacturers have targeted their R&D efforts towards creating better sensors. Mature and ubiquitous sensors, e.g., for temperature, movement, light, have gone through improvement loops increasing accuracy, reliability, robustness, or cost-effectiveness. Obviously, making better sensors is a good business strategy for a sensor manufacturer. However, when applying sensors in practice, a smarter path is feasible.
What if you could get the same signal or – more importantly – the same intelligence with less accurate, reliable, or robust sensors but at a much lower cost, by enhancing the signal collection and post-processing with AI? This feels counter-intuitive, but using low-fidelity sensors and AI sensors has proven to create good-enough results with an order of magnitude lower cost for an installed base.
This is the basic idea that MOOS applies towards creating a sensing surface to assess inventory positions and movements 'in the field', such as in shelves, drawers, or other stock-keeping locations.
Boosting the cost-benefit ratio
There are several pathways in which AI can improve the performance of a sensor that collectively can help boost the cost-benefit ratio. These include the following:
- Taking out gold-plated specs: Good is good enough. In the case of MOOS, the most value is captured in sensing an inventory 'direction', like 'running low', instead of actual product recognition and count in every situation. Playing with this requirement can unlock a superior trade-off between cost and capability.
- Improving signal to noise with AI: Many disturbances are well understood and can be assessed and corrected. For instance, paper-based sensors are highly sensitive to humidity, temperature, curvature, the structure of the support surface, electrical routing, matrix ghosting, and read-out logic. All create variance that can be assessed and corrected for. The same logic applies to mechanical sensors, like long-term drift or non-linearity in force sensors.
- Smart interpretation: With calibrated and repeatable sensors, a rule-based or algorithmic approach for a read-out can be fine. Where there is some degree of variance or interpretation, ML techniques can help uncover patterns and improve interpretations. At MOOS, this applies to interpreting a heatmap of readings across a matrix of pressure cells to recognize products. AI can interpret a series of moving grey dots much better than any human to identify a placement or movements of products. Smart sampling: If similar insights can be realized with fewer sensors and fewer measurements, then the capex and operating cost can go down. Smart combination of different sensors (synthetic sensors), smart sampling of where and when readings are needed, or cut-back on the type of sensors can all help this equation. At MOOS, this is particularly relevant to 'measure what is needed, not what is possible'. The engine that MOOS operates is sensor and signal agnostic. This allows a combination of smart and simple sensors as the situation calls for. Measuring many different small bottles might require a sensor shelf with a high-density matrix, while measuring a stack of paper may simply require a scale.
Potential to rethink sensors
The introduction of adding an AI-layer on top of traditional sensors creates potential performance boost that can not only be used for lower-cost fit-for-purpose solutions, like MOOS does but also push the performance envelope at the higher end. You can just imagine how impactful the path of virtual or synthetic sensors could become. Broader application of this idea might disrupt traditional sensor manufacturers – or relegate them to tier-1 supplier for broader systems.
MOOS capitalizes upon these ideas in creating connected shelves. Lowering the cost of the installed base can unlock a broader series of use-cases and open application areas that were previously unattractive in terms of pay-back time.